In the ever-evolving landscape of libraries, the challenge of consistent and accurate title matching across cataloging practices has become increasingly pronounced. This challenge can impact a variety of library functions – from managing diverse resource types and formats (print, electronic, digital, or books, video, audio and more) to resource sharing, resulting in time-intensive manual problem-solving on the backend and frustration for library users on the frontend.
At Ex Libris, part of Clarivate, we have decades of experience in harnessing the power of burgeoning technology to lead a transformation in library experiences. Now we’re working closely with our trusted community to develop a groundbreaking AI solution poised to revolutionize title matching, providing a unified approach that supports all library activities.
We understand the limitations of the current system. Books are cataloged differently by content providers as well as librarians in various institutions, and sometimes even within the same institution. Existing methods for title-matching fall short due to an inability to determine the quality of matches, disparate criteria for matching, and non-conformity between products.
Our proposed AI solution
Our approach starts with an exact title match, aiming to prevent duplication in resource management and to streamline collection development and resource sharing. Leveraging AI capabilities, our focus is on utilizing models that provide a unified solution for title matching on all platform products, aiding catalogers and librarians in our joint pursuit of accurate and singular cataloging of titles.
The initial steps involved testing different AI models on various record fields to find the optimal fit. Assumptions prioritize high precision over higher recall, with a correct match identified through a matching number. The process includes normalization of author, title, and other fields, followed by selecting the best-performing AI model and determining the most helpful fields for the model.
The breakthrough comes with the inclusion of resource type, normalized title, normalized author, and publication year within a transformer model, that then maps these and delivers the best results for semantic search. While exact matching is a significant stride, the true challenge lies in expanded title matching, encompassing different resource types, editions, works with instances, and more.
Harnessing AI for title matching in libraries opens a realm of possibilities. As we delve deeper into the potential of AI, the goal of a unified approach to title matching for supporting all library activities has the potential for greater efficiency and accuracy, for example in the collection development process and beyond.
This article is the third in our series on AI. Read our previous blog posts on Conversational Discovery and Linked Open Data.
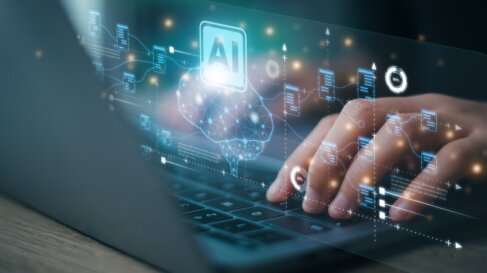
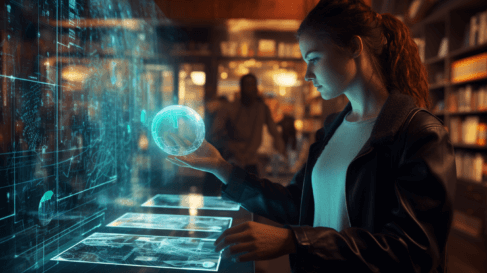
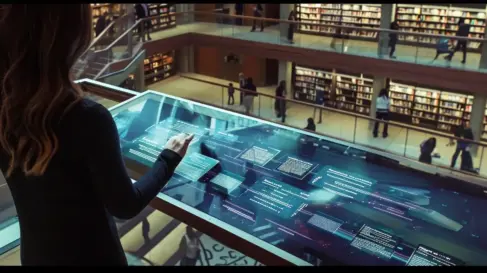
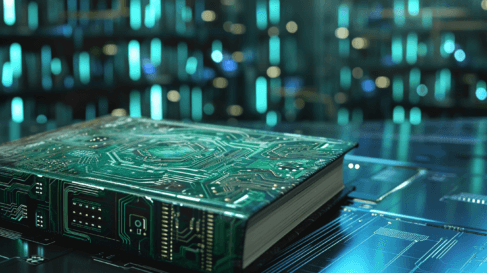
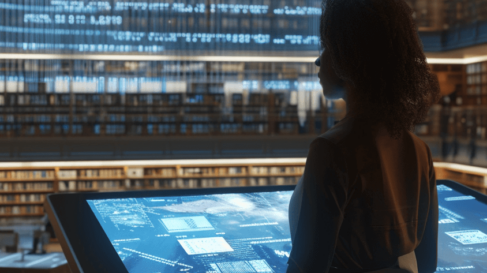